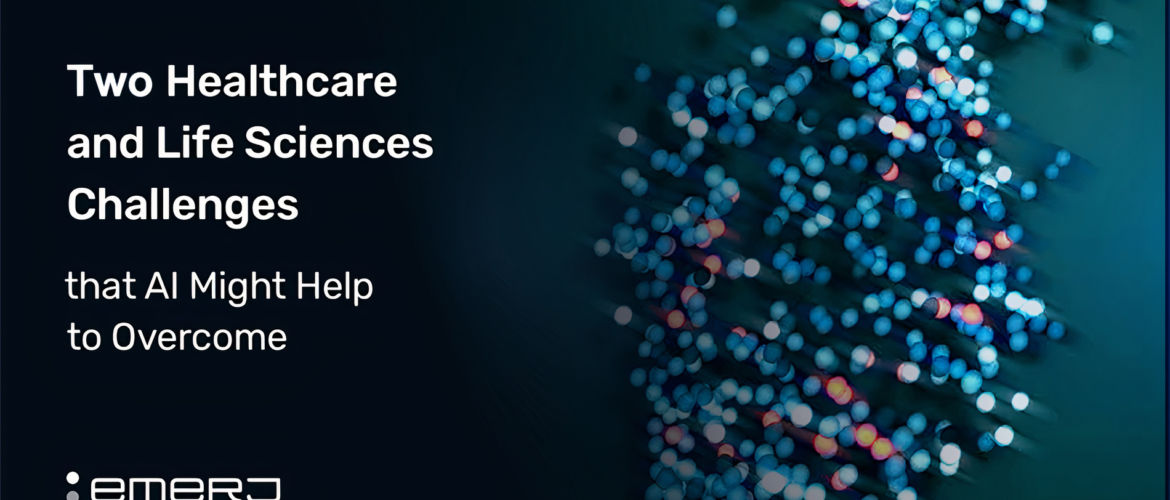
This article was initially written as part of a PDF report sponsored by SambaNova Systems and was written, edited, and published in alignment with our Emerj sponsored content guidelines. Learn more about our thought leadership and content creation services on our Emerj Media Services page.
In the two years since COVID-19, we’ve covered dozens of healthcare and AI uses cases at Emerj – and we’ve seen a major uptick in AI adoption in life sciences (a trend made clear from our interviews with both AI vendors and life sciences AI leaders).
According to the 2020 McKinsey Global Survey, 44% of high-performing healthcare and pharma companies will increase their investment in AI during the COVID-19 crisis—a trend sure to continue as the worth of AI becomes increasingly apparent through the value it brings to today’s healthcare and life sciences sectors.
This week we focus on two distinct use cases:
- AI for Drug Discovery – Creating more useful hypotheses and tests to discover new compounds and make better use of existing compounds.
- AI for Diabetic Retinopathy – Diagnosing a specific and subtle eye disease with computer vision.
We’ll explore what kind of data is used for each application, how data and algorithms come together to solve the problem, and how the workflow changes with AI.
The use cases below come from a white paper we co-created with SambaNova. Many of our initial quotes came from Bill Fox, a healthcare industry leader at the said firm – but the use cases themselves are by no means specific to SambaNova’s technology.
We’ve enriched this and some other white paper materials with outside quotes from Emerj’s other expert interviews. We’ve also added “related resources” to the bottom of both sections below to help interested readers explore these topics in greater depth.
Challenge
Recommendation systems are oft-used in the business world to match consumers with products. The reason for this is that each person is different concerning personal tastes. Similarly, people’s genetic makeup is different – and this is where a recommendation model may be helpful. In this particular case, to “match” individuals with potentially compatible pharmaceuticals.
The stakes are high, as the process of bringing a drug to market is both time and resource-intensive.On average, a new drug takes an average of seven years and $3 billion to develop, from idea to prescription. Many pitfalls await nascent drug ideas. Priorities shift. The drug development process moves too slowly. In the end, two of every three products do not survive to become drugs.
Actions Taken
“There’s a tremendous amount of data and knowledge locked inside an organization,” explains Bill Fox, SambaNova’s Healthcare and Life Sciences Lead. “It’s siloed, and in people’s heads, notebooks, or in a database they created. It’s in different formats. Some are structured. Some aren’t. That’s difficult to pull together.”
It is also apparently difficult to pull together the tremendous amount of data in medical literature, the most voluminous and ubiquitous of which being peer-reviewed journals. Researchers across the various medical disciplines, including pharmaceuticals, use these data to conduct research and construct medical articles of their own. Perhaps one of the most promising use cases of machine learning is to help researchers with this arduous task.
Elaborating on the use of natural language learning in this regard, Amir Saffari, Senior Vice President of AI at BenevolentAI, says:
It’s a fairly complex task trying to harmonize across maybe hundreds of different sources of unstructured data and bring it together. But what you can do is use [unstructured data] to seed natural language processing systems, [and] use those trained AI systems to read literature, extract similar kinds of facts and information, and then pull it together into a form that is servable.
To bring the science of deep learning recommender models (DLRMs) to pharma’s drug discovery process, SambaNova claims they put this data into a model that works like the human brain. The company purports that it built a DLRM that made connections in the data. The DLRM would support the giant drug discovery firms as they navigated the laborious, time-consuming, resource-intensive road that took targets to prescription.
We’ll examine the process below.
Step 1: Knowledge Graphs
- SambaNova claims to have organized the data the way our brains see it—by association. First, project members created a collection of interlinked descriptions of objects, events, and concepts through linking and semantic metadata. This process allowed SambaNova to provide a framework for data integration, unification, analytics, and sharing.
Step 2: Artificial Intelligence
- Next, SambaNova’s NLP team claims to have used PyTorch to define and train the biomedical text-mining algorithms to find the right data and patterns and trends in that data. The team then fed this data into a knowledge graph. The knowledge graph scrutinized the data like a human scientist would, by looking for connections between genes, proteins, diseases, and compounds.
Step 3: Informed Recommender Models
- SambaNova then claims to have used the knowledge graph to train the DLRM to find the best targets in the drug discovery pipeline.
Results
“If you can turn a two-year process into two months or three weeks,” reflects Fox, “how far could you go with an AI-powered recommender model before you need to move to animal or human trials? How much can you speed up those first missteps? Improve the success rate?”
DLRMs and knowledge graphs potentially speed up the drug discovery process. They also potentially enable AI to make new connections, discover new pathways, and test ideas without incurring the traditional costs of experimentation. The concept behind SambaNova’s AI-informed recommender models appears to be to enable the collaborative efforts of hundreds of researchers – and decades of experience and observations—to be in the digital room, opining on the viability of new targets.
SambaNova’s DLRM solutions appear to allow the pharma industry to identify viable drug discovery targets faster and more efficiently across multiple application scenarios. With AI adoption accelerating in pharma, SambaNova seems to be well-positioned to help the drug discovery process players navigate the challenges of bringing these technologies to the next level.
Related Emerj Resources
- Article: AI at Johnson & Johnson – Current Investments
- Interview: The Future of Pharma Data, AI, and Drug Development – with Glen de Vries of Medidata
Use Case 2: Diabetic Retinopathy
Challenge
The world’s population is aging. Experts predict that healthcare resources may become scarce as Gen Xers and millennials reach their retirement years by mid-century. UN data indicates that 16% of people alive in 2050 will be older than 65, up from 11% today. And, by the end of the 2020s, the world will face a shortage of some 15 million healthcare workers.
“Developing countries will feel those shortages even more,” says Bill Fox.
During that same time, the prevalence of diabetic retinopathy is expected to increase nearly 100% to some 14.6 million people in the United States alone. Already, diabetic retinopathy ranks as the most common diabetic eye disease in the US and is a leading cause of blindness in adults.
One of the potentially-impactful uses of computer vision (CV) is detecting and diagnosing diabetic retinopathy in a way that is both more economical and democratic.
SambaNova sought to find a CV solution that provided accurate, effective diagnoses of diabetic retinopathy in patients—one that allowed them access to earlier screenings and that enabled healthcare facilities to maximize the efficient resourcing of their healthcare personnel.
Actions Taken
In an attempt to develop a CV solution that would help resource-strapped healthcare professionals detect diabetic retinopathy in patients, SambaNova built an AI model and taught it what to look for in patient images. The company appears to emphasize the importance of precision in detecting minuscule anomalies.
We are hearing more use cases where CV is utilized to detect tiny objects in medical imaging and diagnostics. Previously on our podcast, Yufeng Deng, Chief Scientist at Infervision, talked about how CV is being used to detect lung nodules, a potential indicator of early lung cancer:
Lung nodules are tiny three to four centimeters-round things that could develop into lung cancer later on. They’re small, hard to find, and typically radiologists have to look at several images several times and with different views to localize [them].
In a similar way, SambaNova claims it built a recommender model that uses Neural Network (NN) reasoning. “We want the Neural Networks (NN) to see the eye the way a human brain sees and processes it. AI must see the whole image like a doctor would,” says Fox.
Regarding the model’s attributes, SambaNova claims to have prioritized ease of use, accuracy, efficiency, and scale. Concerning the last, SambaNova claims to have built the solution to deliver cellular-level imaging capable of detecting movements as small as 1/100 the size of a strand of a human hair—more than 100 times more sensitive than other eye-tracking systems.
The supposed purpose of the model was first to determine if swelling and leaking blood vessels were present in the eye. If so, decide whether to report a diabetic retinopathy diagnosis to the healthcare professional.
Results
SambaNova’s solution shows that high-resolution computer vision can potentially impact patient outcomes. The company claims that its solution can now detect eye movement at the micron level, significantly improving the standard 2-4mm range available in other systems. Beyond identifying and diagnosing diabetic retinopathy, AI-powered neural networks can potentially extend to identifying and diagnosing different cancer types.
“We use all that data,” says Fox. “It may tell us something we didn’t think of, some insight. It’s unlocking the next generation. We’re moving away from us directing AI and toward AI telling us something we didn’t know or expect.”
The company claims that the model can capture 30,000 data points per ten-second video. It’s also claimed that use cases include the detection and diagnosis of other ailments, including multiple sclerosis and dementia, and have also found applications in pain management.
“For some rare conditions,” Fox adds, “we will see only a few instances in our careers. But, if we can load a couple hundred thousand images, the corpus of knowledge grows.”