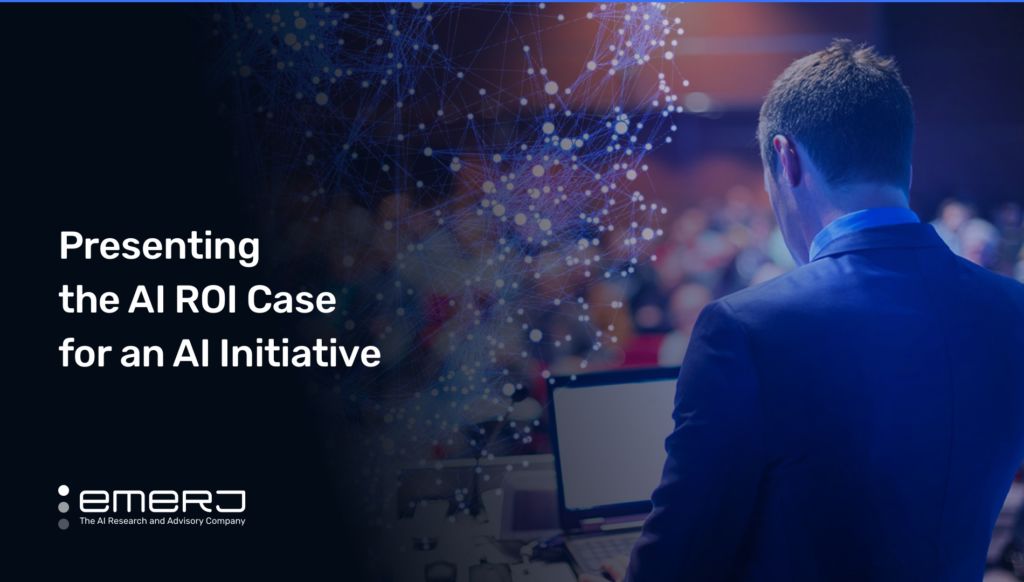
After we’ve constructed a list of potential AI projects, and assessed their ROI and costs, we’re ready to pick the projects that we will take to leadership for approval.
AI project selection requires a company to develop executive AI fluency. However, beyond understanding AI, a company also needs to identify and understand its own AI maturity level.
This understanding will inform decisions regarding which projects to move forward toward the decision and commitment stage, and even whether it’s the right time to move forward with initial AI projects at all. This decision point is particularly relevant for smaller firms with little to no in-house data science talent – or firms with pressing near-term financial problems.
That said, if you believe a set of AI projects is worth presenting to leadership, the basic outline below should provide a structure that will come in handy for conveying AI’s value.
Final Project Prioritization
In some cases, it makes sense to present AI projects as substantial transformation initiatives. In others, it may make sense to present more modest and limited AI projects – with relatively little emphasis on long-term transformation.
Choosing the best path forward for your company depends on several factors:
For firms that have fewer resources for AI, less executive AI fluency, and less in-house AI talent: It often makes sense to posit small AI projects, designed to deliver results, build basic AI maturity and confidence, and generate lessons learned for leadership and subject-matter experts.
For firms with more resources for AI, more executive AI fluency, and more in-house AI talent: It often makes sense to present a larger portfolio of AI projects, with a broader, more transformative vision, and potentially more complex and transformation-oriented (versus purely near-term value-oriented) projects.
Genuinely transformative AI projects (i.e. projects that require significant R&D budgets, more in-house talent, and more AI understanding and context) should only be presented to leadership if they can consider those factors.
AI champions in smaller firms run the risk of scaring the buying stakeholder or getting the stakeholder to naively commit to projects that they are unable to deliver on. As an informed AI champion, it’s your responsibility to present the right amount, number, and size of AI projects based on the readiness of your organization and its decision-makers.
Presentation Order
While there is no single magic slide deck to guarantee that you’ll win budget resources for your AI project, there are presentation structures that work, and presentation structures that do not.
The objective of this section isn’t to create an ossified system that all presentations must follow, but to highlight the elements of a successful AI business case. A basic best practice structure is presented below:
- Frame Strategic ROI
- Introduce the Application Itself
- Provide Social Proof and Examples
- Show Measurable ROI
- Talk about Costs
- Show Capability ROI
- Show Strategic ROI Before and After Story
Let’s explore these steps in order:
1. Frame Strategic ROI
When presenting an AI project to leadership, it’s important to lead with the higher-order strategic goals that the stakeholder in question (whoever the economic buyer is) cares most about.
This process is simple when leadership is explicitly interested in AI for strategic purposes – but this is rarely the case.
Most of the time, the AI champion (presumably, you, the reader of this report) bears the burden of framing the strategic value of AI. The way that framing happens depends on the circumstance:
- When a buying stakeholder is explicitly interested in solving a specific problem (e.g., reducing call center expenses). In this case, we can refer to the higher-order strategic goal that makes that near-term goal important. Knowledge of the firm’s strategic goals and a few rounds of “why” questions can often get this job done. If a stakeholder has asked us to improve inventory estimates, we might frame our proposed AI solution as a way to achieve a related, higher-order strategic goal of the company. For example, if one of the firm’s strategic goals (or one of the stakeholder’s strategic mandates) is to improve gross margin, an AI initiative around logistics and supply chain prediction might be framed as an investment in that deeper profit margin goal.
- When a buying stakeholder is interested in AI for its own sake (i.e. “We should use AI for customer service”). A “toy” AI application is a project done for the sake of AI itself, not for any reasonable near-term benefit, and not with any tie to either building AI maturity or achieving strategic outcomes. When a buying stakeholder has an interest in AI, but no rational strategic goal for AI initiatives, an AI champion should focus first on clarifying the aims of the project, and the near and long-term goals that the stakeholder is interested in. Champions who ride on “AI interest” alone set themselves up for failure, as AI projects inevitably require resources, the time and attention of team members, and new ways of working – all of which come across as a surprise (and so often result in projects being canceled) to buying stakeholders who decided to pull the trigger on an ill-informed toy project.
- When a buying stakeholder has no initial interest in AI or a specific problem to solve. In this case, we need to lead with the strategic goals that are generally most important to the stakeholder, and bring up the AI initiative as a bolstering force to support those longer-term aims. Any suggested portfolio of AI projects should generally be filtered by both (a) what would be most useful in reaching company goals and driving value, and (b) what is most strategically relevant and pressing in the mind of the buying stakeholder(s).
The long-term success of many AI projects rests on the ability to move beyond unrealistic short-term financial gains, and into a broader understanding of AI’s role in transforming a firm and bringing it closer to its longer-term strategic goals.
Skipping this step entirely is an error, and sets up buyers with unrealistically optimistic expectations for AI as a plug-and-play solution, as opposed to an iterative and new way of working.
We explore the importance of leading with strategy in our Emerj Plus article titled: How to Succeed with AI Projects – Lead with Strategy.
2. Introduce the Application Itself
After we frame the strategic aim of the company or buying stakeholder, we can explain the actual AI solution we’re proposing.
Buying stakeholders usually have little interest in the technical nuances of the solution. It’s most important to convey the following factors (below), and we suggest doing so in the following order:
- What will be the business output of the solution? (i.e., What results and outputs will this solution deliver?)
- How will workflows change for our team members? (i.e., Will new software and tools be used?)
- What resources will the project require? (e.g., time, money, data, etc.)
If possible, simple images or short videos of the proposed AI solution should be used to make the application clear.
Use the “closed eyes test.” Explain your AI solution to a non-technical friend or family member, in the same way that you plan to present it to your buying stakeholder – and do so in under 2 minutes. If your friend or family member can’t clearly “see” the application in their mind in a way that makes sense, then assume that your buying stakeholder won’t be able to, either.
3. Provide Social Proof and Examples
Examples of similar AI applications used by similar companies help to make an AI application understandable. These examples also help to prove the value of this kind of AI application to skeptical stakeholders.
General tips to bear in mind about using examples:
- It’s always most convincing to talk about examples that involve companies of a similar size, and/or of companies in the same industry.
- Whenever possible, use examples of named companies using this kind of AI solution (e.g., “Coca-Cola used X kind of computer vision solution to automate Y inventory process”), rather than anonymized vendor examples (e.g., “A global pharmaceutical firm used our solution to…”).
Whenever possible, find examples that involve directly referenced results. Ideally, these results should be presented in a format that could be directly extrapolated to apply to your own goals.
For example, if you find a use-case of a competitor using a kind of AI solution to improve eCommerce cart value by 12% over 6 months, you can show what the same bump in improvement would result in within your organization. Or, if a firm used an AI solution to reduce the manual time required to maintain a type of manufacturing equipment by 40%, you could determine the amount of time and money being used on manual maintenance in your own business, and demonstrate what a 40% reduction would mean in both time and money. This plug-and-play example helps to drive home the potential value of an AI solution.
Whenever possible, it makes sense to reference direct competitors who are using a similar AI application similarly. Enterprise firms are notoriously mindful of what their direct competitors are doing.
This can (and often does) mislead enterprise leadership to follow blindly in the footsteps of competitors’ AI press releases, even if those highly touted AI solutions are delivering little or no value (this is exactly what happened with banks and chatbots). Use social proof to bolster the argument for AI projects that are likely to deliver value – as social proof is often among the most powerful forces influencing executive decision-making.
4. Show Measurable ROI
Present your measurable ROI in the form of revenue driven, costs saved, or risks reduced.
Again, it is best to use tangible, plug-and-play examples when presenting measurable ROI. While it’s possible to use hypothetical examples and estimates of improvements, it’s hard to beat the impact of having real references from similar companies in similar industries.
5. Talk about Costs
Just as AI champions shouldn’t estimate the cost of AI projects until they know that the project has sufficient strategic and near-term value – stakeholders will care little about the costs of an AI initiative until they believe it’s worth a potential investment. Presenting costs after strategic value and measurable ROI, then, is the right order.
It should be emphasized that cost estimates will never be accurate. Instead, cost estimates serve the purpose of differentiating orders of magnitude.
Tertiary cost estimations (even if well-informed by data scientists and subject matter experts) may not be able to tell us if one AI project is slightly more or less costly or time-consuming than another – but they should be able to show us which AI projects are likely to be vastly more time-consuming, or resource-intensive, than others.
6. Show Capability ROI
The costs of AI projects typically involve elements of AI maturity (again, refer to Emerj’s Critical Capabilities model of AI maturity). An AI-powered payment fraud solution might require major changes to data infrastructure and significant hiring efforts for AI talent. A chatbot might involve years of cross-functional collaboration between data scientists and customer support professionals.
After presenting the costs of an AI solution, we recommend that an AI champion present the benefits of building AI capabilities into the process.
Any given AI solution has several what we might call “AI maturity opportunities” hidden underneath its costs and challenges. It’s not important to find all of them.
Rather, it’s important to address the key challenges to adoption (which could be costs, cultural changes, skills requirements, etc.) of the AI project that you are recommending, and framing the AI maturity opportunities that could be hidden within each of them – along with the strategic benefit of building those elements to maturity.
This is arguably the only way to present the challenges of AI adoption truthfully without scaring away buying stakeholders from potential AI projects. Again, making a sale based on a buyer’s unrealistic and naive assumptions about easy near-term ROI is the most common way that AI projects get set up to fail.
Capability ROI isn’t something leadership will be thinking about when they hear a pitch about AI projects, but it’s something they need to understand – even if only at a light and conceptual level – if they’re to commit to AI investments that pay off.
7. Show Strategic ROI Before-and-After Story
A presentation design to win resources and approval for an AI project should not only convey the costs and benefits of AI, but it should also put those costs and benefits in the context of a firm’s current position.
We recommend summing up a suggested AI application with an understanding of where the firm is today, what kind of actions and steps would be required to bring the AI project to life, and what the “after” picture would look like.
Ask: What processes will be changed, and how? What old costs should ideally be reduced? What new costs and processes will be added?
These tactical, real-world changes should be framed – at the beginning of the presentation – under the umbrella of strategic objectives.
For example:
We are not only reducing expenses associated with customer support chat agents, but we are also setting ourselves up to improve our margins and improve our customer experience in the long run.
We are not only improving the cart value of new visitors to our eCommerce page, but we’re also taking steps to win mindshare of our most important buyer segments, and building more marketing resources that we can use to achieve our 5-year market share goals.
Projects that are not couched in strategic value run the risk of promising too much by way of near-term results, without conveying enough long-term value for stakeholders to continue funding the AI project once it runs up against hurdles and challenges (which is inevitable).
Other Presentation Considerations
Structure Your Presentation Around Stakeholder Priorities
Prioritize the AI applications you present – and all elements of your presentation – based on the priorities of the buying stakeholders.
As much as possible, emphasize this fact in the presentation by directly referencing the stated goals of the firm, or, even better, of the buying stakeholders themselves. Taking excellent notes and asking excellent questions early-on in the AI project selection process means having more ammunition for alignment with your buyer when presentation time comes.
Multiple Presentations for Different Stakeholder Groups
Be prepared to have multiple versions of your presentation, appealing to the strategic value and “before-and-after” story of the particular stakeholder group you’re presenting to.
Within a healthcare system, getting buy-in from hospital CEOs will be very different than getting buy-in from doctors. The workflows and costs and benefits will impact them differently. Doctors may take the flak from confused and disgruntled nurses and office staff who are learning to use a new software tool – while the CEO may mostly be concerned about reducing costs while maintaining or improving patient outcomes.
Parting Advice: Frame the Long-Term Value of AI
Implementing AI represents a journey. Take leadership along for the ride, but make sure they know where they’re going – and how many resources they can expect to invest before they arrive at your intended destination.
Be frank when you talk about near-term and long-term value. Help them see the measurable results AI will bring. Build a strong foundation for your AI project and the projects that will follow.
Present your AI ROI case for your AI initiative. Educate leadership about the strategic, long-term value of AI and avoid the pitfall of framing AI as a plug-and-play tool that delivers only short-term financial ROI.
To help you prepare your AI ROI presentation, check out Emerj’s whitepaper, Achieving Sustainable AI Adoption with the AI Framing Scale. The AI Framing Scale helps AI leaders and external AI consultants frame the strategy and long-term nature of AI adoption when you communicate with executive leadership.